Detection of Alzheimer's Disease in MRI Images Using Deep Learning and Saliency Map - دانشکده فنی و مهندسی
Detection of Alzheimer's Disease in MRI Images Using Deep Learning and Saliency Map
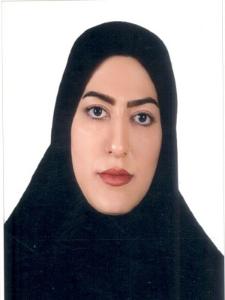
نوع: Type: thesis
مقطع: Segment: masters
عنوان: Title: Detection of Alzheimer's Disease in MRI Images Using Deep Learning and Saliency Map
ارائه دهنده: Provider: samira mostafapour
اساتید راهنما: Supervisors: Dr. Hassan Khotanlou
اساتید مشاور: Advisory Professors:
اساتید ممتحن یا داور: Examining professors or referees: Dr.mirhossein dezfoulian - Dr.moharam mansoori zadeh
زمان و تاریخ ارائه: Time and date of presentation: 2022
مکان ارائه: Place of presentation: salon khan mohammadi
چکیده: Abstract: Alzheimer's disease is a degenerative disease in which the volume of the brain decreases, and the brain neurons and their connections are destroyed. This disease leads to disorders in patients' behavior, memory and reasoning. So far, there is no definitive cure for Alzheimer's disease, but if the disease is detected in the early stages and treatment begins from the early stages of the disease, the effectiveness of treatment methods will increase and will have a greater impact on the patient's treatment process. Brain imaging techniques are one of the most effective tools in diagnosing Alzheimer's. So far, many computer-aided diagnostic methods have been proposed in the field of Alzheimer's disease, which can be used to diagnose Alzheimer's disease by receiving medical images of the patient's brain and performing image processing. In this study, a deep learning-based approach for automatic detection of Alzheimer's from brain CTscan images is presented. The proposed approach includes preprocessing steps for format conversion and noise reduction, saliency map for feature extraction, color mapping for converting images from 2D to 3D, and finally convolutional neural network. In this study, three different saliency maps and four different architectures for the convolutional neural network segment have been used, which has led to the development of twelve different models for the diagnosis of Alzheimer's. Evaluation of the proposed methods on one hundred CT scan images from the Oasis dataset, considering 20% of the data for the test, 8% for the validation, 72% for the training, led to different results on the twelve developed models. The calculated accuracy, precision, sensitivity,and AUC criteria for all models were between 63% and 76%, and the best model was obtained using CovSal saliency map and ZFNet convolutional neural network resulted in 73% accuracy, 73% accuracy, 73% sensitivity, and 76% AUC. All comparisons of the proposed methods with the base paper showed that almost all of the proposed methods work better than the baseline and produce more reliable results
فایل: ّFile: Download فایل