Hybrid Model Based Reinforcement Learning for Energy Management in Heating Ventilation and Air Conditioning (HVAC) Systems - دانشکده فنی و مهندسی
Hybrid Model Based Reinforcement Learning for Energy Management in Heating Ventilation and Air Conditioning (HVAC) Systems
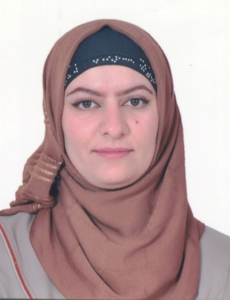
نوع: Type: thesis
مقطع: Segment: PHD
عنوان: Title: Hybrid Model Based Reinforcement Learning for Energy Management in Heating Ventilation and Air Conditioning (HVAC) Systems
ارائه دهنده: Provider: Suroor Moaid Dawood Al-Salih
اساتید راهنما: Supervisors: Alireza-Hatami and Raad Z. Homod
اساتید مشاور: Advisory Professors:
اساتید ممتحن یا داور: Examining professors or referees: Mohammed Hassan Moradi, Kahdim Zarraa& Mohammed Abidini
زمان و تاریخ ارائه: Time and date of presentation: 26/6/2022 , 12-14
مکان ارائه: Place of presentation: Class 10
چکیده: Abstract: The main objective of Heating, Ventilation, and Air Conditioning (HVAC) control system is to reduce energy consumption while providing comfortable indoor environment with the optimal levels. Different methods for modeling the HVAC have been discussed in this thesis. Furthermore, a comprehensive literature survey on the HVAC systems control methods has been presented. Moreover, the control approaches are classified and the benefits, drawbacks, and key features of each are extracted. The Machine Learning (ML) algorithms used in HVAC systems to control the indoor temperature and/or CO2 concentration levels of buildings while minimizing energy consumption, energy costs, power grid peak load, and providing ancillary services like frequency regulation have been reviewed. A modified model of the HVAC system has been developed and verified, and its performances are compared with those of residential load factor (RLF) model with and without the Takagi–Sugeno Fuzzy (TSF) controller. The results of the proposed model and the RLF model are compared in different ways, demonstrating that the proposed model is more efficient and stable than the RLF double cooling coil model, with energy-saving around 10.06 %. The energy consumption reduction, retaining the levels of indoor air quality and thermal comfort of the users are two significant factors to consider when evaluating the new work environment. Therefore, a novel HVAC system considering the temperature and CO2 concentration as continuous states in an integrated model has been developed using energy conservation laws and Lagrange polynomials modeling based on mass conservation law. Also, model-based (MB) Reinforcement Learning (RL) online architecture that takes optimal decisions for on/off HVAC system, lighting, open/close doors/windows system, and fresh/return air dampers ratios for creating an intelligent work environment has been presented and applied on the new HVAC system model. At each time step, the control system receives outdoor/indoor temperatures and CO2 concentration, and then responds with the best control actions that can provide the desired users' comfort levels. The deterministic policy (DP) has been matched with a model-based reinforcement learning (MB-RL) method to provide a DP-MB-RL technique to analyze the system's performance in terms of the building occupants' comfort levels and energy savings. However, for optimizing the HVAC system control, the deep cascade feed forward (CF) and then the nonlinear autoregressive exogenous (NARX) neural networks (NNs) have been used as approximation functions for the DP-MB-RL control method. The hybrid deep CF–RL and deep NARX-RL control approaches combine the CF and NARX advantages, such as high prediction, functions approximation, and generalization with optimum decision making advantage that is useful for RL. The improved algorithms for the DP-MB-RL network structures, the hybrid DDP-CF-RL, and DDP-NARX-RL, show good performance of tracking the indoor temperature and CO2 concentration levels compared to the MB-RL approach. But the DDP-NARX-RL method yields the best performance as compared with the other three control methods due to its ability to identify the continuous state-action spaces and massive data training in the shortest time. Also, the HVAC system performance is improved without any fluctuations, overshoot/undershoot, and disturbance elimination. In addition, the energy consumptions of a building, by applying four methods, such as MB-RL, DP-MB-RL, DDP-CF-RL, and DDP-NARX-RL along a day are evaluated. The results showed that the DDP-NARX-RL achieves energy savings up to 20.5% as compared with MB-RL method and 6.5 compared to DP-MB-RL approach. Also, the results are remarkable, as by applying the DDP-CF-RL and DP-MB-RL method, the energy consumption of the building along a day is reduced by 17.25% and 15.031% respectively, as compared with MB-RL. In addition, the system's energy costs for the four controllers have been calculated and evaluated based on different electricity pricing schemes and the system cooling coil loads. The results prove that the DDP-NARAX-RL controller has a better performance than the others as the system runs with lowering electricity costs at the lowest Fixed pricing (FP), Time of use (TOU) pricing, and Real-time pricing (RTP) schemes. Furthermore, the DP-NARX-RL is applied for controlling a multi-chiller HVAC system for 24 hrs, and its performance is compared with the RL and PID controllers from different aspects such as thermal comfort conditions, energy consumption, and energy cost for various pricing schemes. The results show the better performance of the proposed controller compared with benchmark controllers. MATLAB Simulink software program is used for simulation tasks of the building, HVAC system model, and the different controllers
فایل: ّFile: Download فایل