Multi-model Assembly Line Balancing with Learning and Forgetting Effects of Workers - دانشکده فنی و مهندسی
Multi-model Assembly Line Balancing with Learning and Forgetting Effects of Workers
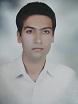
نوع: Type: thesis
مقطع: Segment: masters
عنوان: Title: Multi-model Assembly Line Balancing with Learning and Forgetting Effects of Workers
ارائه دهنده: Provider: Ahmad Faridanifar
اساتید راهنما: Supervisors: Parvaneh Samouei (Ph.D)
اساتید مشاور: Advisory Professors:
اساتید ممتحن یا داور: Examining professors or referees: Javad Behnamian (Ph.D) - Hamid Reza Dezfoolian (Ph.D)
زمان و تاریخ ارائه: Time and date of presentation: November, 15 , 2020
مکان ارائه: Place of presentation:
چکیده: Abstract: One of the subjects that manufacturers are involved with, is the issue of diversity of customers' tastes, in order to manage this situation in such a way as to produce products with minimal change, they need to consider so-called multiple lines that have the necessary flexibility to produce these products. On the other hand, lots of products need assembly operations. Hence, in this article, two new mathematical models for Multi-model Assembly Line Balancing have been introduced that assembly operations have been done by workers manually. But, in order to more accurate scheduling, differences between workers from the view of learning and forgetting effects on assembly line balancing have been intended. The objective of this article is to minimize number of work stations so that, it can help produce diverse products for covering different taste of customers with decreasing in constructing station, hiring and employing manpower indirectly. In the first model, all parameters consider certain. In the second model, because customer's demand may not be constant, and also this factor can affect cycle time, a robust approach has been used to solve in three different scenarios. Also, due to the fact some problems with this model in medium and large dimensions are not able to solve in rational time with commercial optimization software like GAMS, therefore it provides the condition of using meta-heuristic algorithms. In this research, proposed algorithms are Particle Swarm Optimization (PSO) and Krill Herd Algorithm (KHA). Then, after solving problems in small dimension with GAMS and also solving medium and large dimensions ones with mentioned algorithms, analyzing on several problems show that robust modeling provides more confident design and allow to decision-makers (DM) to have better assembling based on better understanding of short-term and long-term conditions under conditions of demand uncertainty. Also regarding to obtained results, KHA algorithm achieves to near optimum solution than another algorithm in much less solving time, and it shows the power and efficiency of this algorithm in solving complicated mathematical problems. Keywords: Assembly Line Balancing, Multi-model Assembly Line, Learning and Forgetting Effect, Particle Swarm Optimization Algorithm, Krill herd Optimization Algorithm
فایل: ّFile: Download فایل