Predicting neonatal mortality based on risk factors using data mining techniques - دانشکده فنی و مهندسی
Predicting neonatal mortality based on risk factors using data mining techniques
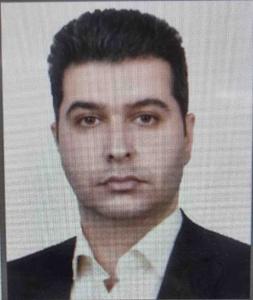
نوع: Type: thesis
مقطع: Segment: masters
عنوان: Title: Predicting neonatal mortality based on risk factors using data mining techniques
ارائه دهنده: Provider: Mohsen Hemmati
اساتید راهنما: Supervisors: dr.Hamidreza Dezfolian
اساتید مشاور: Advisory Professors: dr.Mohammad Heydarzade
اساتید ممتحن یا داور: Examining professors or referees: dr.Vahid Khodakarami-dr.Amir saman Kheyrkhah
زمان و تاریخ ارائه: Time and date of presentation: 12:30pm 2024/01/23
مکان ارائه: Place of presentation: Industrial Engineering Seminar
چکیده: Abstract: The death of neonates is always one of the concerns of families, the medical community and the relevant officials of every country. Neonates are the future builders of their country, and the loss of every neonatal entail significant financial and life costs for that country. Predicting the death of neonates is a very important issue, and today, with the help of data mining techniques, this can be done with high accuracy and the number of deaths of these human capitals can be reduced. The purpose of this research was to predict the death of neonates using data mining techniques, and for this purpose, birth data related to March 2021 to September 2021 of the Iman system of the Ministry of Health was used. First, among the 68 variables, the outcome variable of childbirth was considered as the target variable, and by using the methods of Feature selection like Chi-Squared, Information Gain, Correlation-Based Feature Selection, Fast Correlation-Based Filter, and Sequential Feature selection(Forward Selection and Backward Selection), Recursive Feature Elimination for Support Vector Machine and Least Absolute Shrinkage and Selection Operator, 8 more important features were selected to perform the data-mining process. Then, to implement the classification technique, 7 Naive Bayes methods, K-Nearest Neighbors, Support Vector Machine, Decision Tree, Random Forest and Gradient Boosting were used, among which the Gradient Boosting method with values of 0.995 ,0.9978, 0.9760 and 0.9769 performed the best for accuracy, precision, recall, F1 score and AUC criteria, respectively. In the next step, by using clustering methods including Agglomerative Clustering, K-means Clustering, DBSCAN Clustering and Clique Clustering, the data is divided into different clusters. Among these methods, the K-means clustering method performed best with values of 0.2616 and 1.26, respectively, for the criteria of Silhouette and Davies Bouldin index, as well as the clustering of dead cases into 5 clusters. In the last step, using each of the Apriori methods and FP growth, which are among the methods of association rules, 50 rules were obtained, of which 84% of the Apriori rules and 96% of the FP growth rules led to the results of zero 5-minute Apgar score and the use of forceps or vacuum in interventions during childbirth. The collection of these results can be used to help doctors and relevant officials to predict the death of neonates and reduce the cost of life and money. Key Words: Neonatal mortality prediction, Feature selection, Classification, clustering, Association rules
فایل: ّFile: Download فایل