Evolutionary RNN-Based Human Activity Recognition in Smart Places - دانشکده فنی و مهندسی
Evolutionary RNN-Based Human Activity Recognition in Smart Places
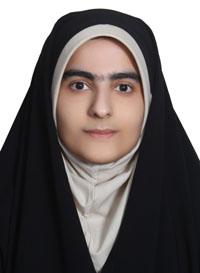
نوع: Type: thesis
مقطع: Segment: masters
عنوان: Title: Evolutionary RNN-Based Human Activity Recognition in Smart Places
ارائه دهنده: Provider: Mohaddeseh Bidy
اساتید راهنما: Supervisors: Dr. Muharram Mansoori zadeh
اساتید مشاور: Advisory Professors:
اساتید ممتحن یا داور: Examining professors or referees: Dr. Hassan Khotanlou - Dr. Reza Mohammadi
زمان و تاریخ ارائه: Time and date of presentation: Saturday 03.13.2021
مکان ارائه: Place of presentation:
چکیده: Abstract: To increase quality of life and control available and using resources of people, considering advance of ambient assisted living technology, we need human activity recognition in smart environments. these environments include internet of things sensors. To designe intelligence internet of things systems we need data recorded from existing sensors of these environments analysis with artificial intelligence knowledge algorithms. Human activity recognition in smart environments means analysis of data from existing sensors by artificial intelligence knowledge. There are different algorithms for activity recognition. Traditional machine learning algorithms provided hard and time consuming methods in both feature extraction and network architecture definition. With appearence of deep learning methods provided extract features automatically. Althogh evolutionary algorithms methods provided automatically network parameter definition. Finally with combination of evolutionary algorithms and deep learning a method were provided tackled both automatically feature extraction and network parameter definition so well. But challenge of network architecture definition still remained. In this regard we provide a method with combination of genetic evolutionary algorithm and deep recurrent network. The proposed method is as follow that we define recurrent layer type, recurrent layer numbers and neuron numbers of each layer with genetic algorithm. We evaluate our proposed method on three benchmark datasets of DFOG, UCI-HAD and UCI-Opp and we saw better resaults on two datasets and above 95 percent accuracy on the other. Considering the use of wearable, objective and ambient sensors, challenge of use of data with large volume and with different sensors arrengment and numeros residents were still remained not resolved. In regard of solving this challenge in addition of three benchmark datasets we used uci repository released version of famous CASAS dataset. Our proposed approach shows considerable improvment on this dataset in comparison with previous methods. Also our method reported results in less layer namber and neuron numbers of each layer for every for datasets. This caused running time and model parameter reducing. To designe powerful AIoT system, our proposed approach in addition of current activity prediction, predicts next activity and current and next activity time duration properly. Key Words: human activity recognition, smart environmens, sensor based data, internet of things, recurrent neural network, genetic algorithm, intelligent internet of things.
فایل: ّFile: Download فایل