Improving the diagnosis and classification of retinopathy in retinal images by developing deep networks - دانشکده فنی و مهندسی
Improving the diagnosis and classification of retinopathy in retinal images by developing deep networks
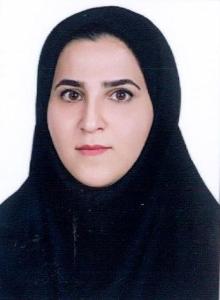
نوع: Type: thesis
مقطع: Segment: masters
عنوان: Title: Improving the diagnosis and classification of retinopathy in retinal images by developing deep networks
ارائه دهنده: Provider: azam afshari
اساتید راهنما: Supervisors: Dr. Hassan Khotanlou , Dr. Muharram Manosoori Zade
اساتید مشاور: Advisory Professors:
اساتید ممتحن یا داور: Examining professors or referees: Dr. afrasiabi , Dr. nosrati
زمان و تاریخ ارائه: Time and date of presentation: 2024
مکان ارائه: Place of presentation: Faculty of Engineering
چکیده: Abstract: Diabetic retinopathy, one of the most common complications of diabetes, manifests early with symptoms in the retina and ranks among the primary causes of blindness. Early diagnosis of retinopathy is crucial as it facilitates prompt treatment and prevents progression and complications such as blurred vision and blindness. This study introduces a deep learning approach for diagnosing and grading stages of diabetic retinopathy using retinal (fundus) images. Our model employs a deep convolutional neural network named ConvNeXtBase, which is pretrained and updated, to extract necessary features and carefully adjust them on a new, comprehensive dataset that includes the publicly available APTOS 2019 dataset. This model has not only demonstrated excellent performance in diagnosing and classifying stages of diabetic retinopathy on the APTOS 2019 dataset but has also shown superior results compared to previous methods. The proposed method serves as a reliable tool for screening and grading stages of diabetic retinopathy, enhancing clinical decision-making and patient care. This research specifically addresses the classification of images into five classes, which is the most difficult and challenging aspect among other classifications. Finally, the performance of the proposed method was evaluated on 20% of the APTOS dataset, achieving an accuracy of 88.92% and an F1 score of 88.95% in classifying the five classes, indicating the successful performance of this model in this task.
فایل: ّFile: Download فایل