Increasing the accuracy of electricity load forecasting in the smart grid by clustering consumers - دانشکده فنی و مهندسی
Increasing the accuracy of electricity load forecasting in the smart grid by clustering consumers
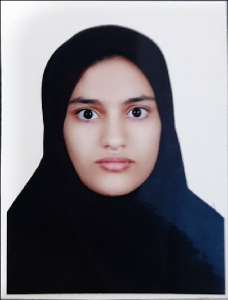
نوع: Type: thesis
مقطع: Segment: masters
عنوان: Title: Increasing the accuracy of electricity load forecasting in the smart grid by clustering consumers
ارائه دهنده: Provider: yasaman maleki
اساتید راهنما: Supervisors: Dr. Morteza Yousef Sanati
اساتید مشاور: Advisory Professors:
اساتید ممتحن یا داور: Examining professors or referees: Dr. Sakhai Nia and Dr. Nosrati
زمان و تاریخ ارائه: Time and date of presentation: 2024
مکان ارائه: Place of presentation: Faculty of Engineering
چکیده: Abstract: Electricity load consumption prediction is one of the most important tools for managing smart grids. It can improve performance (by enabling timely decision-making), resource management (by optimizing the use of various resources such as energy, network, and computational resources), reduce costs, maintain grid stability, and predict failures in the smart grid. In this research, we use clustering to create groups of consumers with similar consumption patterns, allowing for more accurate predictions of the energy needs of each cluster in the future. First, we calculate the representation of the London electricity consumption data, collected through smart meters, using FeaClip. Then, we identify outliers and cluster the non-outlier representations using the hierarchical clustering algorithm. Finally, we feed the equivalent data streams of the created clusters as input to prediction algorithms. To reduce computational load, re-clustering is only performed when a change in the empirical distribution within the created clusters is detected. This program was implemented using the R programming language in the R-Studio environment. Our proposed approach shows a significant improvement over simple predictions (without clustering) and introduces new capabilities for enhancing prediction accuracy. This approach can be effectively utilized in various applications that require high prediction accuracy and contribute to improving the performance of smart grids.
فایل: ّFile: Download فایل