Medical Visual Question Answering in Liver CT Images using Machine Learning Methods - دانشکده فنی و مهندسی
Medical Visual Question Answering in Liver CT Images using Machine Learning Methods
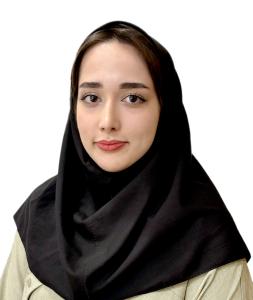
نوع: Type: thesis
مقطع: Segment: masters
عنوان: Title: Medical Visual Question Answering in Liver CT Images using Machine Learning Methods
ارائه دهنده: Provider: Mehrnoosh Chaichi
اساتید راهنما: Supervisors: Dr. Hassan Khotanlou, Dr. Muharram Mansoorizadeh
اساتید مشاور: Advisory Professors:
اساتید ممتحن یا داور: Examining professors or referees: Dr. Mirhossein Dezfoolyan, Dr. Mahlagha Afrasiabi
زمان و تاریخ ارائه: Time and date of presentation: 2024
مکان ارائه: Place of presentation: سالن آمفیتئاتر
چکیده: Abstract: Health has always been one of human concerns, and the use of medical imaging technology greatly aids in the analysis and diagnosis of diseases. Deep learning, as a powerful tool in information processing, plays a significant role in improving health. Some deep learning methods can be used to extract information from images or texts. In this research, a medical visual question answering (VQA) model based on deep learning is presented, specifically designed to analyze liver CT images. This model, utilizing the pre-trained deep neural network architecture ResNet152, is capable of extracting complex features from images. Additionally, BERT is used to convert text words into vectors that encompass the semantic and positional features of the words. The features extracted from images and texts are integrated into a shared space and processed through transformer layers. Each transformer layer includes a multi-head attention mechanism that helps the model better understand the combined information from images and texts. Finally, the resulting vector is fed into a fully connected layer that acts as the final classifier to diagnose and answer liver-related questions. This model can assist doctors and patients in diagnosing and treating liver diseases and improve patients' quality of life. This method has been trained and evaluated on the Liver CT Annotation Image CLEF 2015 dataset, yielding very promising results. Therefore, the use of this model increases the accuracy of diagnosis and ultimately improves the health of patients.
فایل: ّFile: Download فایل