Predicting safe natural childbirth with the aim of reducing risk and treatment costs using data mining techniques - دانشکده فنی و مهندسی
Predicting safe natural childbirth with the aim of reducing risk and treatment costs using data mining techniques
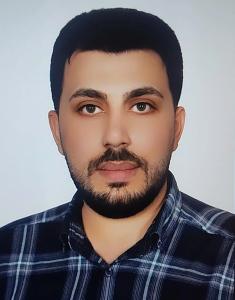
نوع: Type: پایان نامه
مقطع: Segment: کارشناسی ارشد
عنوان: Title: Predicting safe natural childbirth with the aim of reducing risk and treatment costs using data mining techniques
ارائه دهنده: Provider: Ali Nadi
اساتید راهنما: Supervisors: Dr. Nafiseh Soleimani and Dr. Hamidreza Dezfoulian
اساتید مشاور: Advisory Professors: دکتر مرضیه اتوگرا
اساتید ممتحن یا داور: Examining professors or referees: Dr.Vahid Khoda Karami and Dr.Amirsaman Khairkhah
زمان و تاریخ ارائه: Time and date of presentation: 2025
مکان ارائه: Place of presentation: 62
چکیده: Abstract: Safety and health in childbirth are among the fundamental principles of healthcare systems. The objective of safe natural delivery is to provide high-quality services aimed at safeguarding the health of both mother and newborn, reducing potential risks and complications, and enhancing maternal satisfaction. This study examines the concept of risk management in the context of childbirth and its relation to reducing medical expenses, introducing data mining techniques as tools for identifying and predicting risk factors. Data were collected from perinatal and postpartum care by 15 midwifery technicians, cleansed, and analyzed using the CRISP-DM methodology. In this study, three primary risk factors were identified: episiotomy, prolonged labor, and severe hemorrhage. The modeling process was conducted in three approaches: (1) using all variables to evaluate the baseline performance of algorithms, (2) selecting significant features based on expert opinions and modeling with seven data mining algorithms, including Decision Tree, AdaBoost, Bagging, Neural Network, Support Vector Machine, k-Nearest Neighbors, and Naïve Bayes, and (3) applying feature selection criteria to improve predictive accuracy for the risk factor with weaker performance. The results revealed that in the second approach, the three risk factors were modeled, and the algorithms’ accuracy ranged between 71.67% and 97.17%. In the third approach, only the severe hemorrhage factor was modeled, and feature selection improved performance in most algorithms, particularly Bagging and Naïve Bayes, while k-Nearest Neighbors experienced a decline in performance. Predicting and managing these factors could lead to savings of 10 to 15 million IRR per patient and reduce annual costs by 3 to 4.5 billion IRR in public hospitals. This study highlights that promoting safe delivery through data mining techniques has a significant impact on improving family health, reducing healthcare costs, and strengthening the economic and social conditions of society. Reducing treatment-related costs, along with implementing effective policies to encourage natural childbirth and providing higher-quality services in public hospitals, can contribute to sustainable societal development and increase families’ motivation for childbearing. This not only enhances households' quality of life but also plays a critical role in alleviating economic and social pressures on the healthcare system
فایل: ّFile: Download فایل