Writer Identification with Handwriting Analysis using Deep learning - دانشکده فنی و مهندسی
Writer Identification with Handwriting Analysis using Deep learning
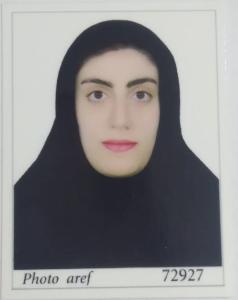
نوع: Type: thesis
مقطع: Segment: Masters
عنوان: Title: Writer Identification with Handwriting Analysis using Deep learning
ارائه دهنده: Provider: Azra solati dalaki
اساتید راهنما: Supervisors: Dr Hassan Khotanlou
اساتید مشاور: Advisory Professors:
اساتید ممتحن یا داور: Examining professors or referees: Dr Muharram Mansourizadeh- Dr Reza Mohammadi
زمان و تاریخ ارائه: Time and date of presentation: Saturday, October 2, 2021
مکان ارائه: Place of presentation: virtually
چکیده: Abstract: Nowadays, many paper documents are converted to digital documents by cameras and scanners. Storage, reconstruction and efficient management of digital documents are important in official automation and digital libraries. As a result, achieving effective algorithms to analyze digital images of documents is a vital need. Writer identification by documents analysis has become one of the interesting challenges in image processing and pattern recognition problems. Identification dependency to handwriting type and structural difference in different people from one hand and quality of images, on the other hand, has made the writer identification problem difficult. So far, various models have been designed for writer identification problems among which deep learning techniques outperform other techniques. In this study, we proposed one model of deep learning based on convolutional neural networks. We used a deep convolution neural network since it has convolution layers to reduce the overfitting risk while helping extract more optimal features from our input images. Our proposed method consists of three steps including preprocessing, feature extraction, and classification. In the preprocessing step, patches are extracted with the same size from different parts of an image in order to keep the size of the inputs uniform and augment the data. Then, to improve the quality of the images, we normalize the images and utilize Gaussian filter to remove noise and Otsu threshold for text separation of background in images. Finally, the obtained images are given to a deep convolutional neural network for the automatic feature extraction. The Convolutional network architecture consists of some convolution layers with three different kernel sizes. In the last step, extracted features are classified by the fully connected layers. Also, in this study, several methods were applied to the CVL dataset for classification and the results were tested and evaluated. The final model is tested and evaluated by different classification measures. The results of the proposed model on the data set demonstrate satisfactory and promising performance